Recently, the group of Image Guided Surgery and Therapy (IGST, Prof. Xin GAO) in Suzhou Institute of Biomedical Engineering and Technology (SIBET) proposed a new radiomic model based on tumor and peritumoral region, which can accurately predict preoperative lymph node metastasis in patients with early non-small cell lung cancer.
Lung cancer has one of the highest mortality rates in the world, and non-small cell lung cancer (NSCLC) accounting for 80-85% of lung cancer patients.
In recent years, the combination of big data analysis technology and medical imaging has brought about the emergence of radiomics method, which is to extract high-throughput information from images for in-depth mining and analysis, so as to achieve non-invasive diagnosis and prognosis prediction of tumors. However, the existing radiomic methods focus on the tumor region and ignore the surrounding environment. Relevant tumor biology studies have shown that the surrounding environment of tumor can secrete a large number of growth factors and cytokines.
Therefore, the extraction and combination the region of tumor and peritumoral features would be expected to provide a new idea for establishing radiomic model and further improve the diagnostic or predictive performance of the model.
The study collected 366 patients with different CT devices. Their radiomic features were extracted from Gross tumor volume (GTV) and Peritumoral volume (PTV) respectively (Fig. 1). Minimum absolute shrinkage and selection operator (LASSO) were used to train the classifier model.
This study showed that the peritumoral features can be used to predict lymph node metastasis in early NSCLC with good classification ability (AUC=0.825). In addition, the model (GPTV, AUC=0.843) established by combining the features of GTV and PTV was superior to the model established by using GTV or PTV features alone (Fig 2.). If the radiomic model and the imaging characteristics of lymph node metastasis were combined to construct the human-machine model, the prediction accuracy would be further improved (AUC=0.862).
The significance of the above research lies in that it verifies the important value of the previously ignored peritumoral region for the prediction of lymph node metastasis, revealing that the man-machine combination mode is a trend for future clinical work and providing a new idea in medical imaging assisted diagnosis.
These works entitled “Can Peritumoral Radiomics Increase the Efficiency of the Prediction for Lymph Node Metastasis in Clinical Stage T1 Lung Adenocarcinoma on CT’’ were published in European Radiology.
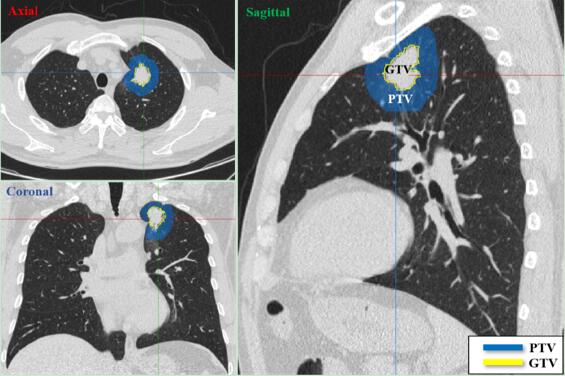
Fig. 1 Two ROIs, GTV and PTV, were defined in axial, coronal, and sagittal CT images
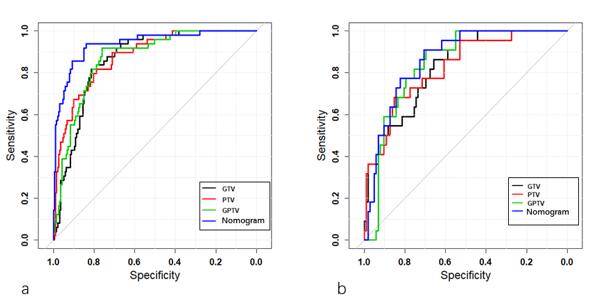
Fig. 2 The receiver operating characteristic (ROC) curves of radiomic signatures: a training cohort, b validation cohort (Image by ZHAO Xingyu)
Contact
XIAO Xintong
Suzhou Institute of Biomedical Engineering and Technology, Chinese Academy of Sciences (http://www.sibet.cas.cn/)
Phone: 86-512-69588013
E-mail: xiaoxt@sibet.ac.cn