Malignant epithelial ovarian tumors (MEOTs) and borderline epithelial ovarian tumors (BEOTs) are two types of ovarian cancer. Accurate preoperative identification to distinguish between MEOT and BEOT will help implement precise treatment, save medical resources, and improve the quality of patients' postoperative life.
Magnetic Resonance Imaging (MRI) has great potentials in distinguishing between MEOT and BEOT. But now, clinical diagnosis highly depends on doctors' experience, which is subjective and of low accuracy.
For the first time, a MRI machine learning-based ovarian cancer diagnosis model has developed to non-invasively identify MEOT and BEOT before surgery by GAO Xin’s research team at the Suzhou Institute of Biomedical Engineering and Technology of Chinese Academy of Sciences in collaboration with JIN Weiqiang from Jinshan Hospital of Fudan University, and other eight 3A hospitals spread across different regions in China.
A total of 501 patients (336 MEOT patients, 165 BEOT patients) were included in the study. Multiparametric MRI images (T2WI FS, DWI, ADC, CE-T1WI) and clinical data (age) were collected. The MRI image features were extracted from the solid component of the tumor and the whole tumor (respectively noted as ST and WT), and a novel machine learning method was implemented to select the features and build a model.
In addition, six board-certified radiologists with different experience and proficiency (employed between 2-13 years) were invited to provide their subjective diagnosis on the cases. The diagnosis performance was further compared between the clinicians and machine learning models.
The results showed that machine learning models can successfully distinguish between MEOT and BEOT (average diagnostic accuracy of 91.7%), compared with the diagnostic performance of six board-certified radiologists (average diagnostic accuracy of 79.5%). The diagnostic ability of the model showed a significant advantage (Figure 1), projecting a more accurate preoperative non-invasive diagnosis of ovarian tumor with the assistance of the esteablished model to clinicians.
This research was mainly funded by the National Natural Science Foundation of China, corresponding results entitled “MRI‐Based Machine Learning for Differentiating Borderline From Malignant Epithelial Ovarian Tumors: A Multicenter Study” were published in the Journal of Magnetic Resonance Imaging.
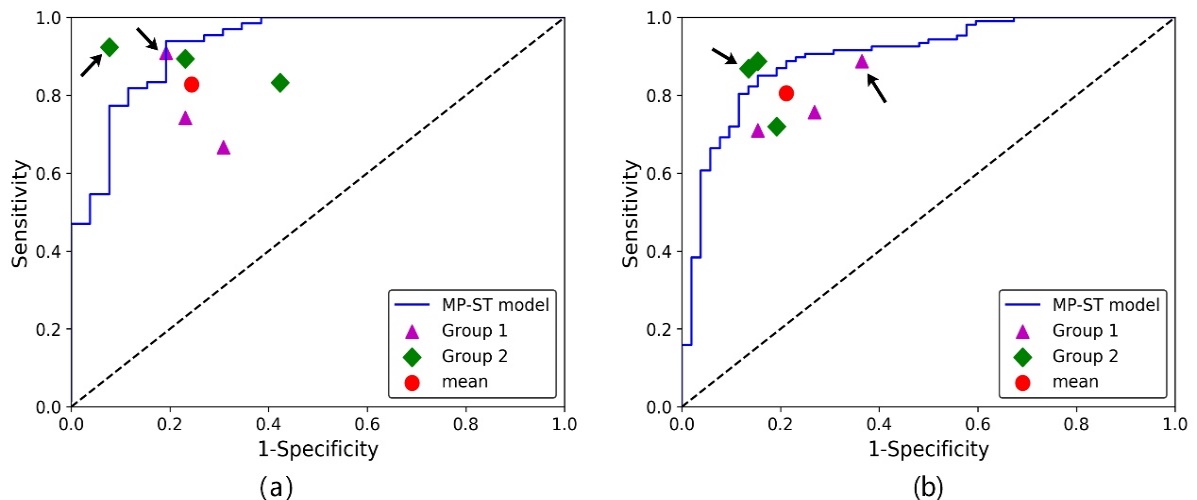
Figure 1. Comparison of diagnostic performance by the machine learning models and radiologists. Purple triangles and green squares represent the diagnostic performance of six radiologists. Red dots represent the average diagnostic performance of six radiologists. Blue line represents the diagnostic performance of the machine learning model. The red dot lying in the bottom right of the blue lines indicates that the diagnostic performance of the machine learning model is better than the average diagnostic performance of six radiologists. (Image by JIAN Junming)
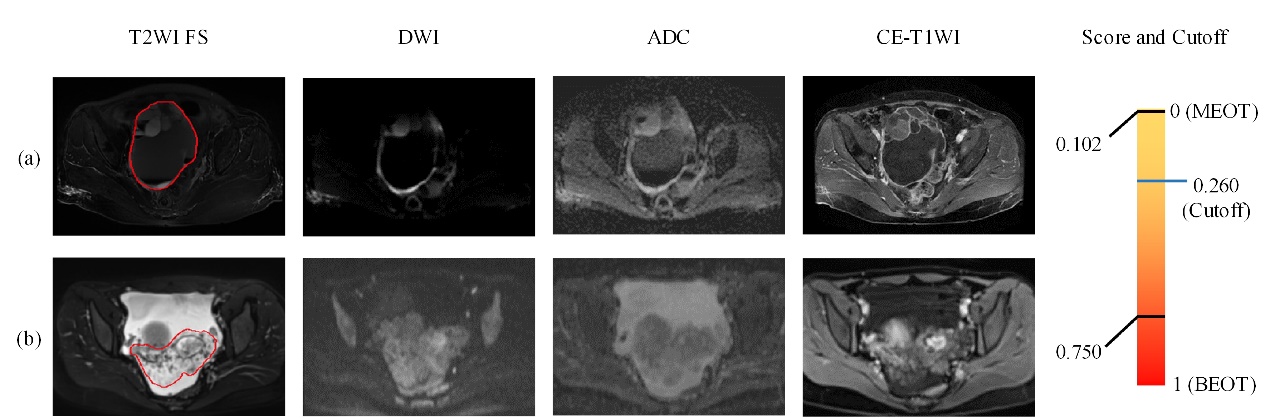
Figure 2: Two cases examples. (a) MEOT; (b) BEOT. The tumor is delineated by the red line. The number to the right of the MR images are the corresponding MP-ST model scores relative to the established cutoff value of 0.260. The diagnosis results are considered to be BEOT when the model score is higher than the cutoff value and to be MEOT when the model score is lower than the cutoff value.. For the first case (pathologic diagnosis of MEOT), the model output score was 0.102. For the second case (pathologic diagnosis of BEOT), the model output was 0.750. (Image by JIAN Junming)
Contact
XIAO Xintong
Suzhou Institute of Biomedical Engineering and Technology, Chinese Academy of Sciences (http://www.sibet.cas.cn/)
Phone: 86-512-69588013
E-mail: xiaoxt@sibet.ac.cn |