Transcatheter arterial chemoembolization (TACE) is the preferred treatment for patients with hepatocellular carcinoma (HCC) in stage B of the Barcelona clinical liver cancer (BCLC) staging system. However, tumor progression occurs in 70%-80% of patients receiving TACE, and these patients have no significant improvement in survival compared with the patients receiving conservative treatments.
The extraction of MRI image features by radiomics is expected to accurately predict the tumor progression after TACE and assist in screening patients with potential benefit from TACE.
An MRI-based radiomics model was developed to realize a preoperative and non-invasive prediction of tumor progression after TACE. This study was done by GAO Xin from the Suzhou Institute of Biomedical Engineering and Technology of Chinese Academy of Sciences in collaboration with LIU Rong from Zhongshan Hospital of Fudan University.
A total of 84 patients were included in this study. Multiparametric MRI data including T2WI, DWI and ADC and clinical information was obtained. The high throughput radiomics features were extracted from tumor area, and a machine learning method was implemented to select features and to build a model.
The results showed that the model predicted tumor progression after TACE with 80% accuracy. The prediction performance of the model combining with multiple MRI sequences was better than that of a single MRI sequence, which indicated that the information contained in different MRI sequences was complementary.
The study suggests that the MRI-based radiomics model has the potential to predict the tumor progression after TACE and to assist in screening patients who may benefit from TACE, thus to help doctors implement precise treatment.
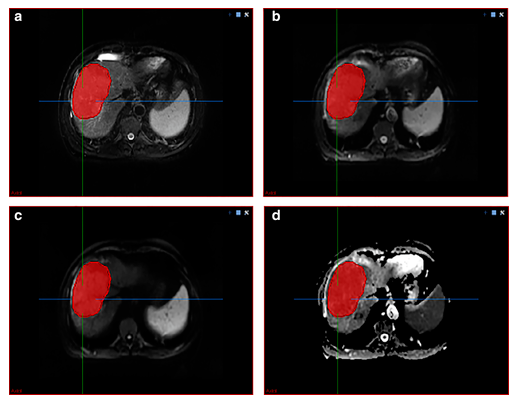
Figure 1.An example of the tumor VOI for different MRI sequences. The VOI was delineated only on T2WI. Other MRI sequences shared the same VOI after being registered to T2WI. (a) T2WI, (b) DWI‐b0, (c) DWI‐b500, (d) ADC. (Image by SIBET)
Contact
XIAO Xintong
Suzhou Institute of Biomedical Engineering and Technology, Chinese Academy of Sciences (http://www.sibet.cas.cn/)
Phone: 86-512-69588013
E-mail: xiaoxt@sibet.ac.cn