Lung cancer is the most lethal cancer in the world. Surgical lobectomy combined with systematic lymph node dissection (LND) is the current preferred treatment for patients with lung cancer.
Accurate prediction of lymph node (LN) metastasis status before operation will avoid invalid LNDs, thus reduce the risk of recurrence and complications, and improve the quality of patients’ life.
A research team led by Professor GAO Xin from the Suzhou Institute of Biomedical Engineering and Technology (SIBET), based on their previous study, proposed a novel multi-scale, multi-task, and multi-label classification network (3M-CN) aiming at the LN metastasis prediction for T1 lung adenocarcinoma patients.
Present image-based preoperative evaluation of LN status for T1 pulmonary adenocarcinoma mainly depends on the judgement of the radiologists. This method is subjective, time-consuming and has a low average accuracy of about 0.7.
The new model, with a backbone of 3D DenseNet, extracts three-dimensional CT features of pulmonary nodules, and fuses different levels of features of pulmonary nodules, with the help of multi-scale feature fusion (MFF) module.
GAO and his team designed a multi-task segmentation module to guide the model to focus more on the nodule region and less on the surrounding structures. This helped realize accurate prediction of LN metastasis risk and multiple related signs evaluation on the basis of multi-label classification task.
Results show that the accuracy of 3M-CN can achieve 0.948, which is the highest in the current reports. The advantage of the proposed method is that the model can predict the LN metastasis without any intervention of doctors, which is fully automated and intelligent, according to GAO.
Meanwhile, the model provides more semantic explanations related to LN metastasis, greatly enhances the interpretability of the deep learning model. It increases doctors' confidence in the model results, and conforms to doctors' diagnostic process for LN metastasis diagnoses in their workflows.
In this study, the CT images and corresponding clinical data from 554 patients with T1 lung adenocarcinoma in two hospitals were used to train and test the proposed network.
The related results were published on Computerized Medical Imaging and Graphics (2021) with the title of "3D multi-scale, multi-task, and multi-label deep learning for prediction of LN metastasis in T1 lung adenocarcinoma patients' CT images".
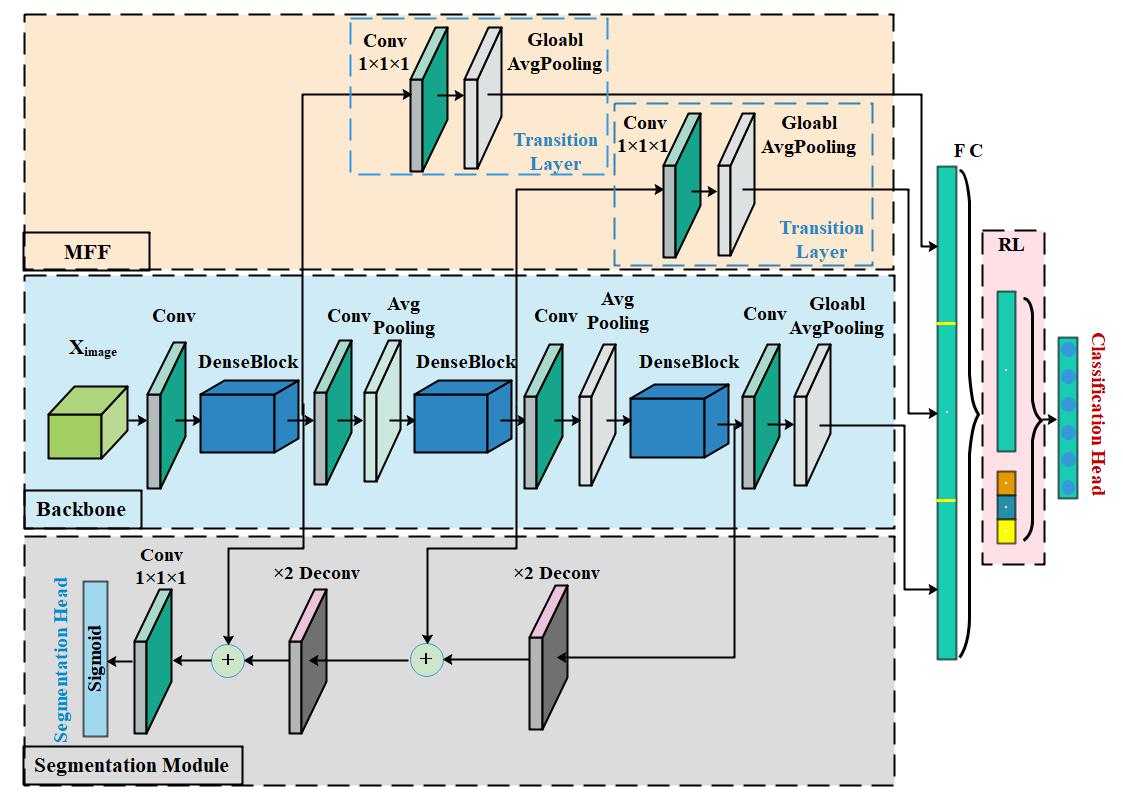
Fig. 1. Architecture of 3M-CN Network. (Image by SIBET)
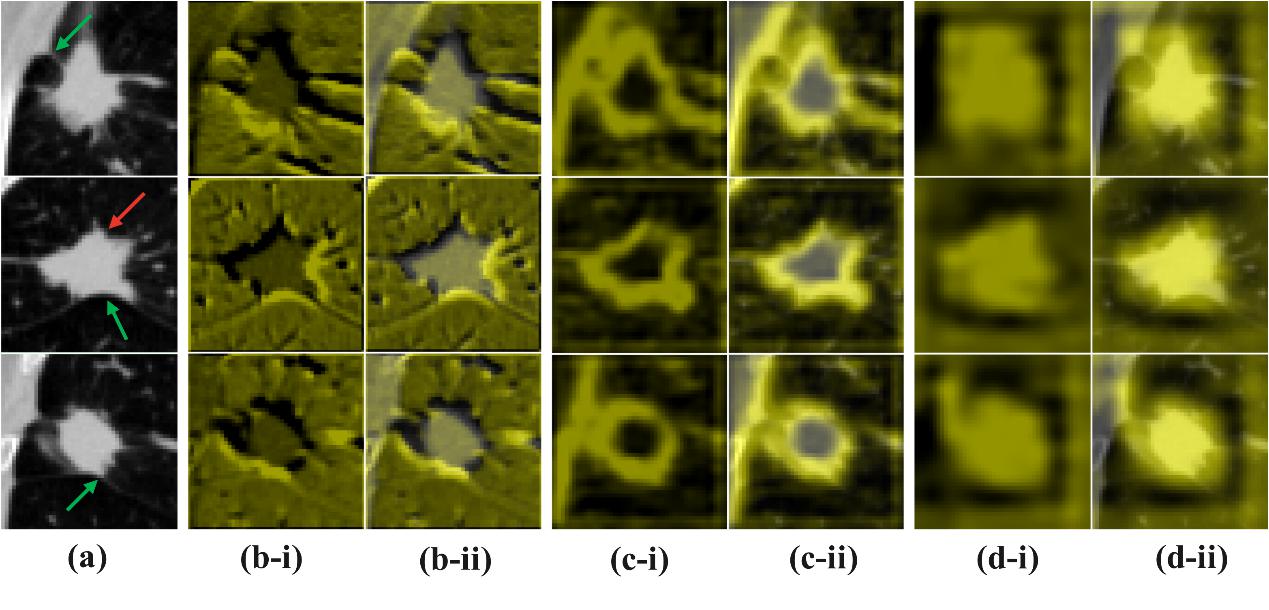
Fig. 2. A case of multiscale feature maps in three views. The figures in the first to third row are axial, sagittal and coronal CT images. (a) The original CT images (red arrow represents lobulation sign; green arrows represent pleural indentation sign); (b-i), (c-i) and (d-i) are the heatmaps interpolated by low, middle and high-level feature map; (b-ii), (c-ii) and (d-ii) are the low, middle and high level heatmaps overlaid on original CT image. (Image by SIBET)
Contact
XIAO Xintong
Suzhou Institute of Biomedical Engineering and Technology, Chinese Academy of Sciences (http://www.sibet.cas.cn/)
Phone: 86-512-69588013
E-mail: xiaoxt@sibet.ac.cn