Effective classification of multi-task motor imagery EEG signals is a way to decode brain's motor intentions, which helps to achieve accurate and efficient multi-dimensional brain-computer interaction. However, the accuracy and robustness of traditional decoding method need further improvement for practical application, due to inherent limitations of low spatial resolution of the EEG signals and the highly difference between the EEG signals of the subjects.
LIU Yan, an associate researcher in DAI Yakang's group at Suzhou Institute of Medical Engineering of the Chinese Academy of Sciences, now proposed a cross-space convolutional neural network (CS-CNN) with customized characteristics method.
“This makes a high-precision and robust multi-task motor imagery EEG signal decoding algorithm possible”, said LIU.
The CS-CNN fuses the global functional representation of the measuring space and the high-resolution brain source activation information of the source space to provide multi-level features. Simultaneously, the customized rhythms and brain regions of interest are screened to realize differential extraction of personalized frequency-spatial valuable information, so as to build the best classification model for each subject and improve the accuracy and robustness of the decoding algorithm as a whole.
Overall block diagram of the CS-CNN is shown in Figure 1.
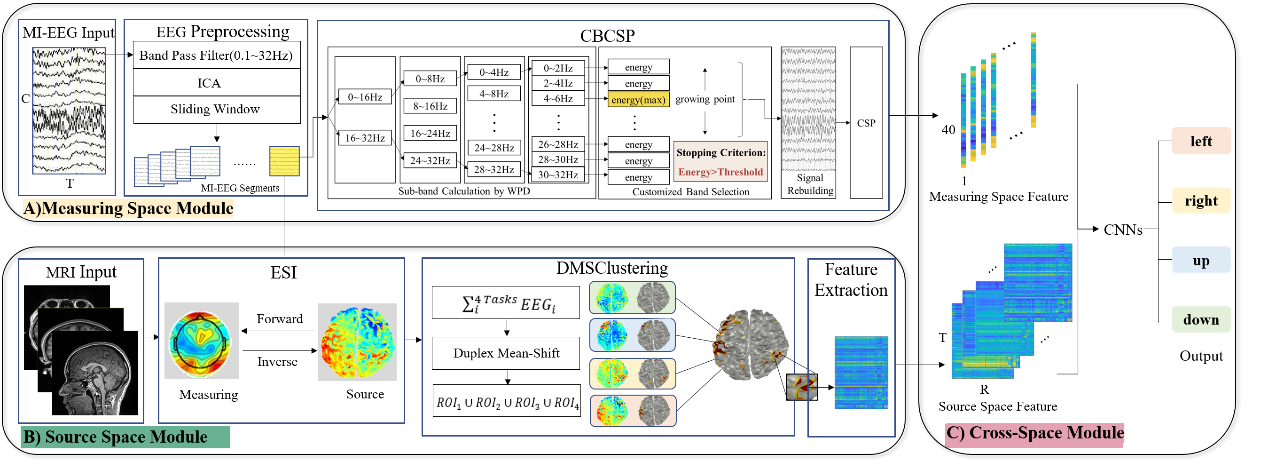
Figure 1. Flow chart of CS-CNN algorithm. (Image by SIBET)
Customized frequency-global spatial feature description is realized through adaptive spectrum sensing and common spatial pattern. Researchers then reversed the EEG signal into the high-resolution source space through the EEG source imaging (ESI), and extracted the high-activation state dipole time series relationship to complete the customized time-high-resolution spatial domain feature description.
LIU and her team extracted the two sets of feature matrices from different spaces and reflecting different levels of information, spliced through convolutional neural network to construct a cross-space global high-resolution customized fusion feature representation, and finally achieved high-precision and strong robust four-category classification.
Validity verification at Suzhou Science and Technology City Hospital showed that the accuracy of the proposed cross-spatial decoding method based on the self-test dataset and the public dataset were 96.05% and 90.37%, and the accuracy range error between subjects is 3.79% and 3.90%, respectively.
“Compared with other state-of-the-art algorithms based on the same public dataset, multiple indicators such as classification accuracy and inter-subject variance have achieved the best performance,” said LIU.
The proposed cross-space method based on customized feature representation can effectively decode multi-task motor imagery EEG information, and provide a reliable algorithm basis for building a multi-instruction set, high-precision and robust brain-computer interface system, LIU said.
Results of the study was published in IEEE Transactions on Neural Systems and Rehabilitation Engineering, entitled “A Cross-Space CNN With Customized Characteristics for Motor Imagery EEG Classification”.
The work was supported in part by the National Nature Science Foundation of China under Grant 62271481 and 61971413, the STI 2030—Major Projects under Grant 2022ZD0208500, Jiangsu Key Research and Development Plan under Grant BE2021012-5 and Grant BE2022049-2, Jiangsu International Cooperation Project under Grant BZ2022028, Soochow Key Basic Research Special Foundation under Grant SJC2022012, Jiangsu Province Basic research project of leading technology under Grant BK20192004.
Contact
XIAO Xintong
Suzhou Institute of Biomedical Engineering and Technology, Chinese Academy of Sciences (http://www.sibet.cas.cn/)
Phone: 86-512-69588013
E-mail: xiaoxt@sibet.ac.cn