Multi-focus image fusion (MFIF) is an image enhancing technology that helps solve the problem caused by depth of field and capture all-in-focus images. It has broad application prospects that can effectively extend the depth-of-field of optical lenses.
Deep learning MFIF methods show advantage over traditional algorithm in recent years. However, more attention was paid to increasingly large and complex network structures, gain modules and loss functions to improve the fusion performance of algorithms.
Now, a team of researchers led by FU Weiwei at Suzhou Institute of Biomedical Engineering and Technology (SIBET) of the Chinese Academy of Sciences reconsidered the image fusion task and modeled it as a conditional generation model.
The team proposed a MFIF algorithm based on the diffusion model, called FusionDiff, by combining the current diffusion model with the best effect in the field of image generation. Their results were published in the latest issue of journal Expert Systems with Applications with the title "FusionDiff: Multifocal Image Fusion Using a Denoising Diffusion Probabilistic Model".
This is the first application of this diffusion model in the field of MFIF, which provides a new way of thinking for the research in this field, according to the researchers.
The principle of image fusion in FusionDiff is shown in Fig 1.
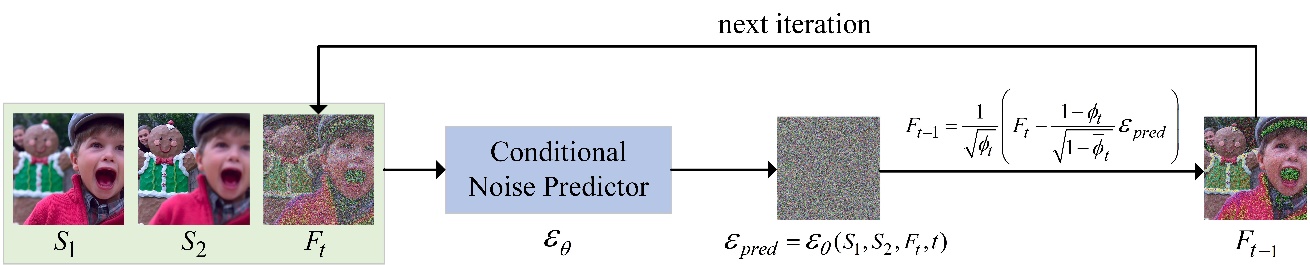
Fig 1. Demonstration of the image fusion principle by FusionDiff. (Image by SIBET)
Experiments demonstrate that FusionDiff outperforms traditional MFIF algorithms in terms of image fusion effect and few-shot learning performance.
"Moreover, FusionDiff is a few-shot learning model, meaning that much effort is not required to produce large-scale datasets,” said FU.
The fusion results obtained by FusionDiff are independent of a large amount of training data, according to FU. “It realizes the transformation from data-driven to model-driven,” he said.
Their study shows that FusionDiff achieve the same quality fusion results as the other algorithms with only 2% of the training data they use. This will significantly reduce the dependence of the fusion model on the dataset, said FU.
A master student, LI Mining, from SIBET, is the first author of this article. PhD student PEI Ronghao is the co-first author and Prof. FU Weiwei is the corresponding author.
This work was supported by the Natural Science Foundation of Shandong Province (ZR2021QE205), and the Youth Innovation Promotion Association of Chinese Academy of Sciences (Y202072).
Contact
XIAO Xintong
Suzhou Institute of Biomedical Engineering and Technology, Chinese Academy of Sciences (http://www.sibet.cas.cn/)
Phone: 86-512-69588013
E-mail: xiaoxt@sibet.ac.cn