Automatic osteosarcoma tumor segmentation on computed tomography (CT) images is a challenging problem, as tumors have large spatial and structural variabilities. In this study, an automatic tumor segmentation method, which was based on a fully convolutional networks with multiple supervised side output layers (MSFCN), was presented.
Image normalization is applied as a pre-processing step for decreasing the differences among images. In the frame of the fully convolutional networks, supervised side output layers were added to three layers in order to guide the multi-scale feature learning as a contracting structure, which was then able to capture both the local and global image features. Multiple feature channels were used in the up-sampling portion to capture more context information, for the assurance of accurate segmentation of the tumor, with low contrast around the soft tissue. The results of all the side outputs were fused to determine the final boundaries of the tumors.
A quantitative comparison of the 405 osteosarcoma manual segmentation results from the CT images showed that the average Dice similarity coefficient (DSC), average sensitivity, average Hammoude distance (HM) and F1-measure were 87.80%, 86.88%, 19.81% and 0.908, respectively. It was determined that, when compared with the other learning-based algorithms (for example, the fully convolution networks (FCN), U-Net method, and holistically-nested edge detection (HED) method), the MSFCN had the best performances in terms of DSC, sensitivity, HM and F1-measure.
The results indicated that the proposed algorithm contributed to the fast and accurate delineation of tumor boundaries, which could potentially assist doctors in making more precise treatment plans.
These works were done by the group of Image Guided Sugery and Therapy (IGST) and published in Computer Methods and Programs in Biomedicine, 143, 67-74 (2017).
Article link:http://www.sciencedirect.com/science/article/pii/S0169260716310926
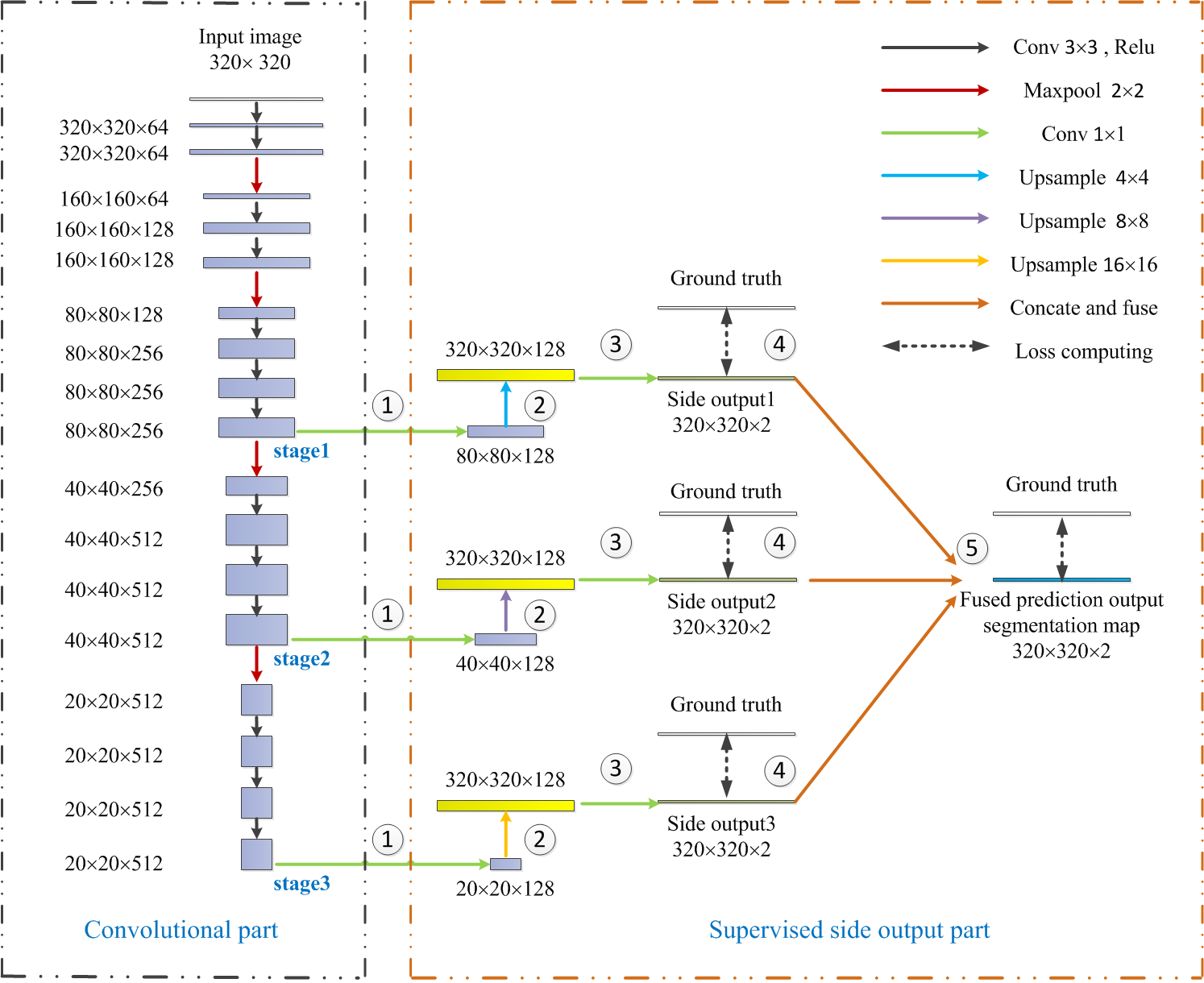
Fig. 1 Architecture of the MSFCN: There were 3 stages with supervised side output layers connected to the convolutional layers. Each side output was guided by a ground truth. A fused layer was added to fuse all the outputs of the side output classifiers.
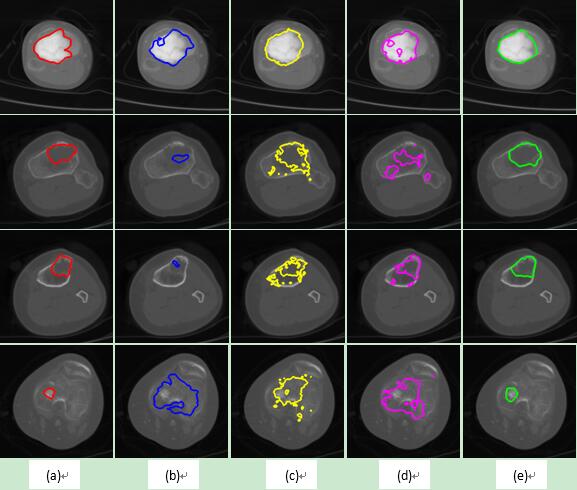
Fig. 2 Segmentation results from the bone lesion osteosarcoma images. Rows 1 to 4 are different cases: (a) ground truth; and (b) to (e) are the results of the FCN, U-Net, HED, and MSFCN methods.
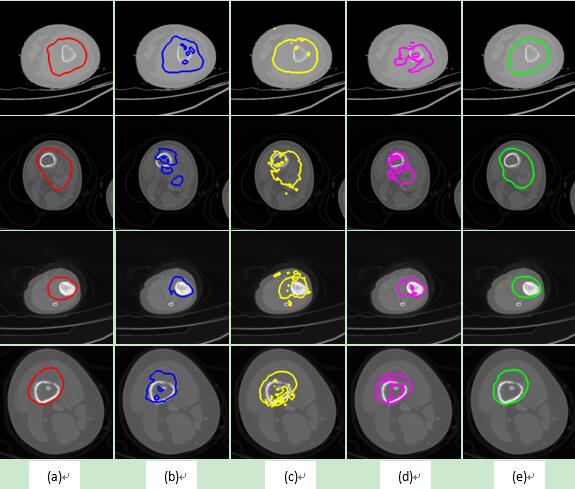
Fig. 3 Segmentation results from the mixed lesion osteosarcoma images. Rows 1 to 4 are different cases: (a) ground truth; and (b) to (e) are the results of the FCN, U-Net, HED, and MSFCN methods.