Multiparametric magnetic resonance imaging (mpMRI) has been accepted as the preferred alternative for rectal cancer diagnosis. N-staging is necessary for all MR reports, for which accurate detection and segmentation of lymph nodes(LNs) are the prerequisites.
However, the bottleneck of current diagnosis methods is the low efficiency of LN identification under the background of multiple organs and multiple intervals. Besides, when the number of slices is more than 20, it will take 3-10 minutes for experienced radiologists to complete the LN detecting of a patient and the results will be affected by the detector's subjective experience, environment, and many other factors: All factors contribute to misdiagnosis.
An intelligent recognition method has been developed and firstly applied to achieve automatic LN detection and segmentation (auto-LNDS) of RC patients before surgery. This development was achieved by GAO Xin’s research team from the Suzhou Institute of Biomedical Engineering and Technology of Chinese Academy of Sciences (SIBET CAS), in collaboration with MENG Xiaochun from Sixth Affiliated Hospital of Sun Yat Sen University, and other three 3A hospitals spread across different regions in China.
373 RC patients were enrolled in this study. The annotations of three senior experts were used as the ground truths for model training. Fused T2-weighted images (T2WI) and diffusion-weighted images (DWI) provided input for the deep learning framework of Mask R-CNN through transfer learning to generate the auto-LNDS model.
The results show that the model has an excellent performance in detecting different scales LNs compared with other reported algorithms (Fig. 1). The size of the detected LNs is smaller (the short diameter is as small as 3mm), and the accuracy is higher, reaching 80.0% detection sensitivity and 0.82 Dice similarity coefficient, which are better than the reported algorithms and junior radiologists (Table 1). In addition, it takes only 1.37s to detect and segment all the LNs of a patient, which is 131 times faster than the doctor's detection speed.
This model could help to minimize differences in LNs detection between radiologists, increase the efficiency of the clinical workflow, and also has the potential to assist physicians in determining the nodal stage. Besides, this method can be extended to the preoperative evaluation of N staging of tumors in various parts of the body based on multimodal images (PET, CT, MRI, etc.), which has a high clinical significance and play an important enlightenment role for automatic identification of LNs in the chest, abdomen and even the whole body.
The research was funded by the National Natural Science Foundation of China. Corresponding results were published as a cover article entitled “Deep learning–based fully automated detection and segmentation of lymph nodes on multiparametric-MRI for rectal cancer: a multicenter study.” in the EBioMedicine (Top Journal of the First District of Chinese Academy of Sciences, published by Lancet).
Table1. Detection Results and Comparison with Other Methods
Method |
Target area |
Scan type |
Nodal Size |
Sens |
PPV |
Time/Vol |
Ours |
Pelvic |
MRI |
≥3.0mm |
80.0% |
73.5% |
1.37sec |
Barbu |
Pelvic+
Aebden |
CT |
>10.0mm |
80.0% |
72.6% |
15-40sec |
Feuerstein |
Mediastinum |
CT |
>1.5mm |
82.1% |
13.3% |
1-6min |
Kitasaka |
Abdomen |
CT |
>5.0mm |
57.0% |
30.3% |
2-3h |
Feulner |
Mediastinum |
CT |
>10.0mm |
65.4% |
52.6% |
135sec |
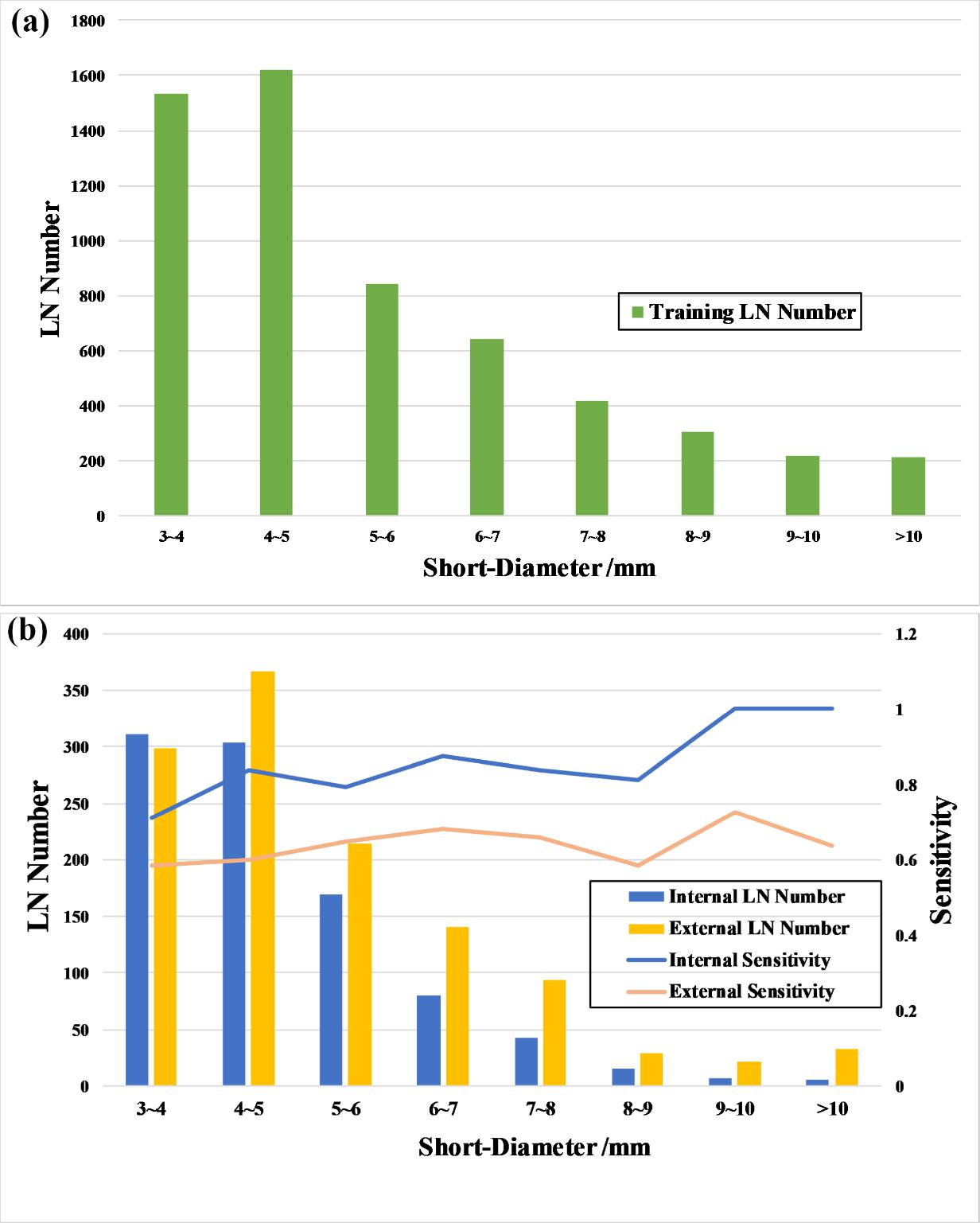
Figure 1. (a) The distribution of LNs short-diameters results in the training dataset; (b) The distribution of LNs short-diameters results in the test datasets; The sensitivity curves of the auto-LNDS model for LNs with different short-diameters in the internal and external test datasets.(Image by SIBET)
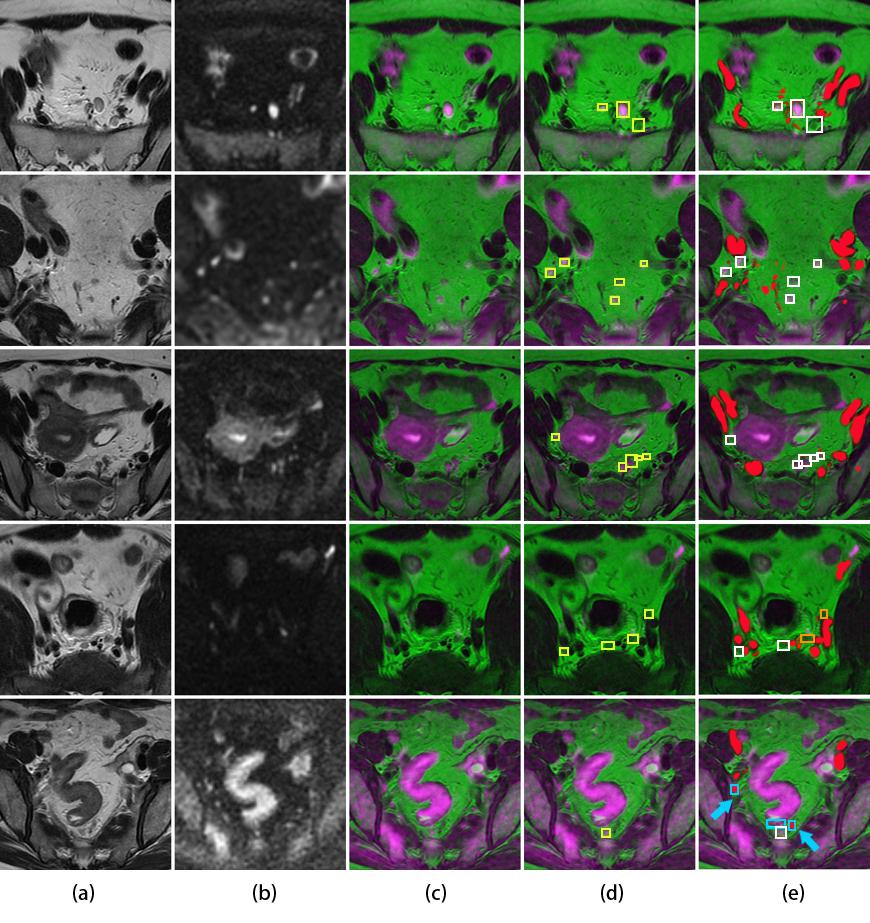
Figure 2. Lymph node detection. a: the original T2WI. b: the original DWI. c: the fusion image. d: the ground truth of annotated lymph nodes with yellow boxes on the fusion image. e: the detected results of auto-LNDS displayed on the fusion images. The white boxes represented the true positives, the cyan boxes represented the false positives and the orange boxes represented the false negatives. Vessels were filled with red. The case in the fourth row shows two missed LNs by the auto-LNDS model. The case of the fifth row, two cyan boxes with red color inside is small vessels misdiagnosed as LNs by the auto-LNDS model (cyan arrow), and the other cyan box is intestinal wall misdiagnosed as LN.(Image by SIBET)
Contact
XIAO Xintong
Suzhou Institute of Biomedical Engineering and Technology, Chinese Academy of Sciences (http://www.sibet.cas.cn/)
Phone: 86-512-69588013
E-mail: xiaoxt@sibet.ac.cn