Scientists at the Suzhou Institute of Biomedical Engineering and Technology (SIBET) have recently developed a machine-learning-based method for early diagnosis of Hirschsprung disease (HSCR) in newborn babies.
This non-invasive method, jointly developed by DAI Yakang’s team at SIBET and HUANG Shungen from Children’s Hospital of Soochow University, will help improve the accuracy of early diagnosis of HSCR in newborn babies.
HSCR ranks the second in congenital gastrointestinal malformation. Delay in diagnosis would increase the risk of Hirschsprung-associated enterocolitis, which represents the leading cause of serious morbidity and death in HSCR patients.
Traditional rectal mucosal biopsy specimen diagnosis method shows high accuracy but should be chosen with great caution for the newly born baby less than four weeks. Clinical examination such as the commonly used barium enema and anorectal manometry, however, sees rather low diagnostic accuracy.
This method developed by DAI’s team based on a combination of clinical and barium enema radiologic features for early diagnosis of HSCR in newborn baby HSCR patients.
The research team extracted quantitative image features from barium enema X-ray films that could effectively depict the morphological differences of intestinal tubes, combined them with expert ratings and common clinical features, and established a prediction model through machine learning algorithm.
This study included 54 newborn baby HSCR patients with biopsy-confirmed HSCR as the cases) and 59 undergoing barium enema for abdominal symptoms but no HSCR as the control.
Colon shape features extracted from barium enema images and clinical features were used to develop diagnostic models using support vector machine (SVM) and L2-regularized logistic regression (LR).
Results showed that the accuracy of human diagnosis based on barium enema X-ray film was 76.99%, while the machine-learning-based diagnosis model achieved 86.36% accuracy, 81.82% sensitivity and 90.91% specificity, and AUC (Area Under Curve) reached 0.9318.
This study demonstrated that a combination of clinical features and colon shape features extracted from barium enemas can help improve early diagnosis of HSCR in newborn baby patients.
The research article “Machine learning-based quantitative analysis of barium enema and clinical features for early diagnosis of short-segment Hirschsprung disease in neonate” has been published in Journal of Pediatric Surgery.
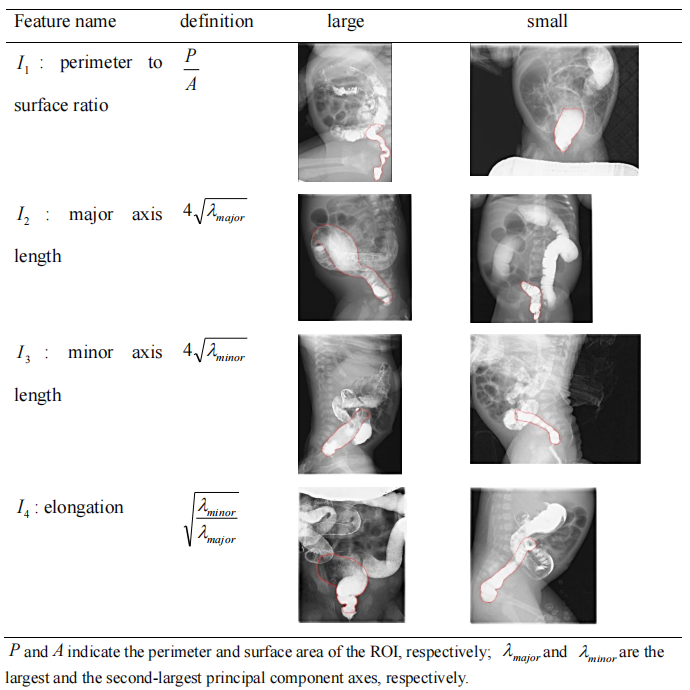
Fig. 1 Colon shape features extracted from barium enema images. (Image by SIBET)
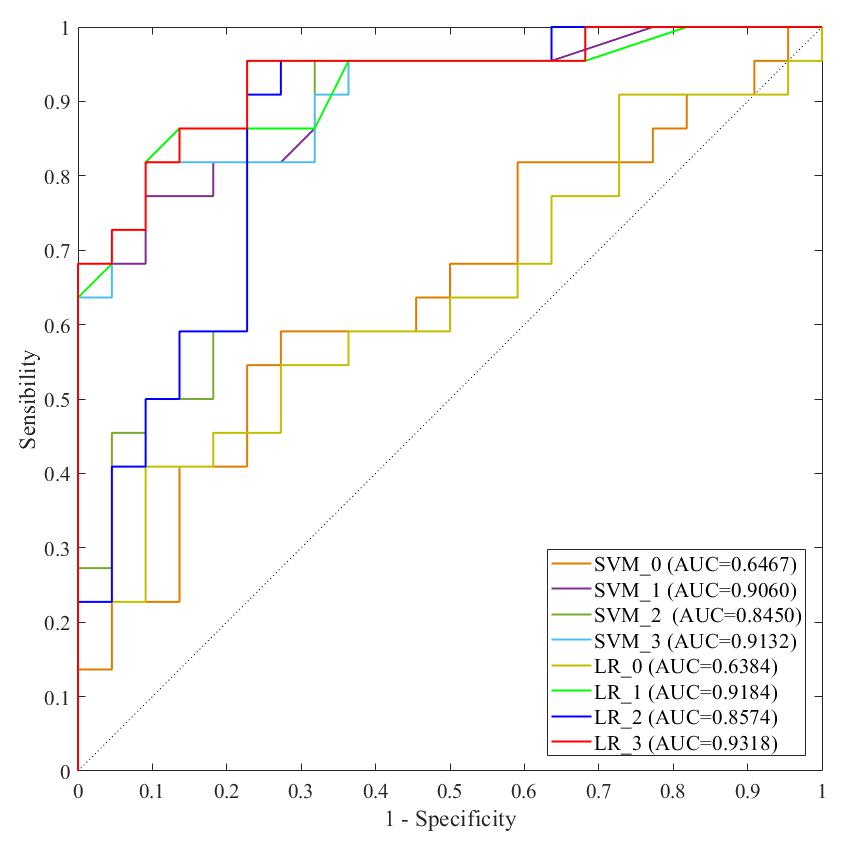
Fig. 2 The ROC curves of SVM and LR classifiers using different feature sets. (Image by SIBET)
Contact
XIAO Xintong
Suzhou Institute of Biomedical Engineering and Technology, Chinese Academy of Sciences (http://www.sibet.cas.cn/)
Phone: 86-512-69588013
E-mail: xiaoxt@sibet.ac.cn